Wound Assessment
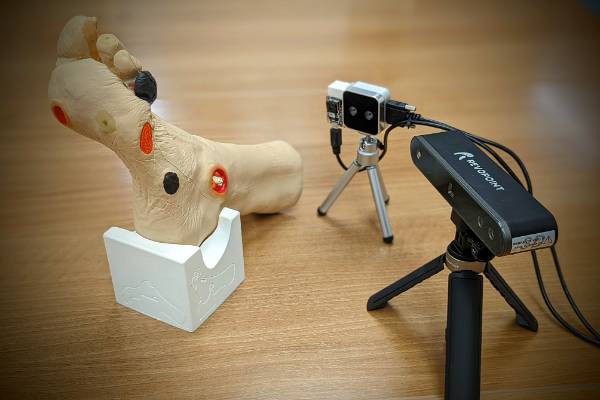
Pressure Ulcers occur due to skin tissue degradation, exposing the layers underneath,
which are very common in diabetic patients. One of the key symptoms shared by many
tissue abnormalities and pathological changes is local temperature change. A multimodal
easy-to-understand 3D visualization of human skin can help clinicians spot problems
immediately and track health progress. Medical thermography is a non-contact and non-invasive
imaging technology that has a variety of clinical applications. 3D thermography is
a cost-effective technology that enhances and improves visual communication, allowing
physicians to look at the temperature of the human body surface from different angles
in a three-dimensional space. This study proposes a low-cost, compact, and portable
3D medical thermography technology. The mobility associated with this technology allows
seamless thermal mapping of a large part of the human body.
A thermal-infrared camera and a depth camera are securely mounted in close proximity
in our system. As a person moves the device around the human body, this system builds
an accurate 3D thermogram model by combining cloud points from each new measurement.
Additionally, our technology benefits from a low-cost thermal sensor, which dramatically
cuts the price.
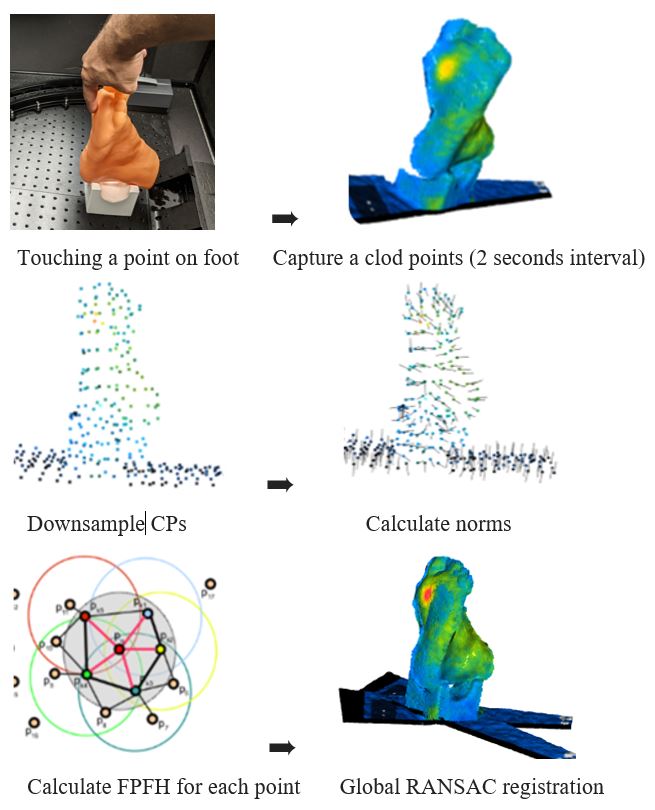
3D reconstruction consists of three steps; RGB-D image construction and cloud point conversion, Fast Point Feature Histograms (FPFH) calculation for each point, and finally the global RANSAC registration [18]. To build a full 3D view around the body part, we need to register multiple cloud points (CP) to each other. It means each CP requires to be registered to the previous one and then merged together. To register CP2 to CP1, we downsample both CPs to increase registration speed. Norms of each point for both CPs will be generated, and Fast Point Feature Histograms (FPFH) will be calculated. FPFH is multidimensional characteristics for 3D CP sets that represent the local geometry surrounding a point. Using Global RANSAC Registration, random points from the CP2 are selected. Their associated points in the CP1 are found by searching the nearest neighbor in the hyper-dimensional FPFH feature space. A rapid pruning algorithm is applied to effectively eliminate false matches between CP2 and CP1. Only points that make it through the pruning stage are utilized to calculate a transformation matrix, which is verified over the entire point cloud. The CP2 will be transformed using the transformation matrix and will be added to the CP1. The final CP1+CP2 will be downsampled to create a new CP1. Fig. 6 shows the diagram for the 3D reconstruction.