Featured Initiatives & Projects
ATIC shares expertise and facility for collaboration on specific focus areas.
Supported by UND's College of Engineering & Mines, research collaborations are expected to create unique engineering research and education capabilities in transportation infrastructure.
Student Design Center (SDC)
The SDC, in partnership with the North Dakota Department of Transportation (NDDOT), the Transportation Technology Research Initiative (TTRI), and UND, puts engineering students to work on real-world transportation designs and engineering issues. SDC interns gain hands-on training and design experience as they work under the direction of NDDOT engineers to prepare studies, plans, and estimates for NDDOT projects. SDC interns are in demand by the NDDOT as well as other agencies, consultants, and other employers as they recognize SDC students have a head start in skills to join the workforce. SDC is conveniently located on-campus, allowing students to work between classes. Additionally, our interns gain unique professional experience to visiting job sites and public hearing meetings to understand the impact of their work as engineers.
UAS for NDDOT
Stockpile Volume Measurement
Rapid and accurate stockpiles volume measurement using Uncrewed Aerial Systems (UAS) equipped with visual imagery. The research team developed this technology coupled with PIX4D software to generate 3D models of stockpiles with 95% and more accuracy.
Engineering Mechanics Institute 2024
Contact
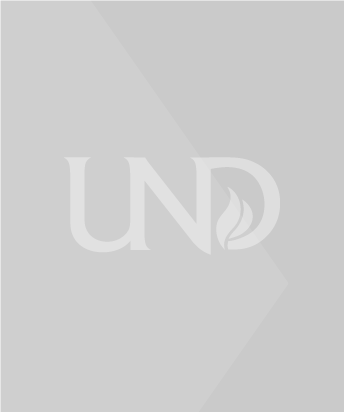
- dalton.l.reitz@UND.edu
- 701.777.3564
-
Upson II Room 260M
Grand Forks ND 58202-8115
Additive Construction
Implementation of autonomy arguably addresses scarcity of civil engineering workforce in Transportation Enterprise in the state of North Dakota. Culverts are underground reinforced or plain concrete structures with backlog in construction. With the support and the enthusiasm received from North Dakota Department of Transportation, additive construction started being investigated by TTRI to develop concrete 3D printing (3DCP) for culvert construction. ATIC is one the pioneers in printing culverts in the world and demonstrated 3DCP for construction of unreinforced pipe culverts.
Publication
Hasani, A., & Dorafshan, S. (2024). Transforming construction? Evaluation of the state of structural 3D concrete printing in research and practice. Construction and Building Materials, 438, 137027.
Presentations
- American Concrete Institute Fall 2023
- American Concrete Institute Fall 2024
- ND ASCE Professional Development Hour
Media
Contact
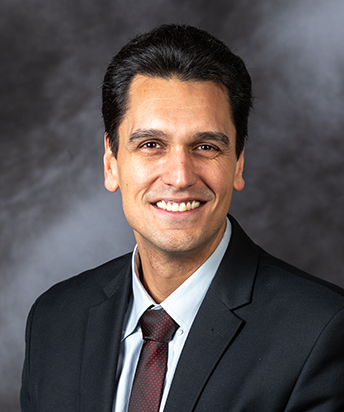
- sattar.dorafshan@UND.edu
- 701.777.0585
-
Upson Hall I Room 218
Grand Forks ND 58202-8115
Periodic inspection of highway ancillary structures plays a vital role in maintaining uninterrupted highway operation. Utilizing small UAS technology allows faster and cheaper inspection; however, the existing available UAS technology and UAS payload does not offer real-time autonomous defect detection using artificial intelligence (AI). A UAS payload was designed for real-time and autonomous defect detection using deep learning models (corrosion, missing bolts, and cracks). A cyber-physical interface is designed to give inspectors control over detection made by AI models.
Publications
- Das, A., Dorafshan, S., & Kaabouch, N. (2024). Autonomous Image-Based Corrosion Detection in Steel Structures Using Deep Learning. Sensors, 24(11), 3630.
- Jafari, F., Dorafshan, S., & Kaabouch, N. (2023, June). Segmentation of fatigue cracks in ancillary steel structures using deep learning convolutional neural networks. In 2023 IEEE/ASME International Conference on Advanced Intelligent Mechatronics (AIM) (pp. 872-877). IEEE.
- Heichel, J., Mitra, R., Jafari, F., Das, A., Dorafshan, S., & Kaabouch, N. (2023, June). A System for Real-Time Display and Interactive Training of Predictive Structural Defect Models Deployed on UAV. In 2023 International Conference on Unmanned Aircraft Systems (ICUAS) (pp. 1221-1225). IEEE.
- Mitra, R., Das, A., Heichel, J., Dorafshan, S., & Kaabouch, N. (2023, May). A Comparative Analysis of Two Deep Learning Neural Networks for Defect Detection in Steel Structures Using UAS. In 2023 IEEE International Conference on Electro Information Technology (eIT) (pp. 430-435). IEEE.
- Das, A., Ichi, E., & Dorafshan, S. (2023). Image-Based Corrosion Detection in Ancillary Structures. Infrastructures, 8(4), 66.
Presentations
Final Report
North Dakota Department of Transportation Report
Contact
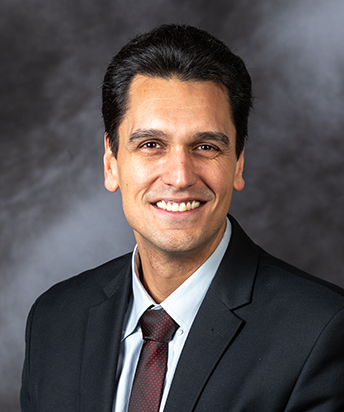
- sattar.dorafshan@UND.edu
- 701.777.0585
-
Upson Hall I Room 218
Grand Forks ND 58202-8115
Distress and deterioration of bridges and large infrastructures is becoming a growing concern. Engineers and researchers are faced with the challenges associated with development and deployment of viable techniques for better management. This has led to adopting advanced Non-destructive Evaluation (NDE) techniques and remote structural evaluation methods in detecting, assessing, and monitoring structural sub-surface defects in bridge decks. However, NDE methods have not been broadly used by bridge stakeholders due to need for skilled operators to interpret NDE for defect detection and bridge evaluation. Recent advances in artificial intelligence can address the limitations; however, development and adaption of the proper artificial intelligence model requires the availability of properly annotated datasets. There exist datasets for surface defects; but due to lack of “ground truth”, datasets for subsurface defects have been limited to laboratory specimens and destructive methods which cannot mimic the reality. In this study, we have developed a reliable annotated dataset designated for subsurface defects for Infrared Thermography (IRT), Ground Penetrating Radar (GPR), and Impact Echo (IE). These data were collected for five in-service Grand forks, North Dakota (ND) bridges that were scheduled for repair and maintenance.
Publications
- Ichi, E., Jafari, F., & Dorafshan, S. (2022). SDNET2021: Annotated NDE Dataset for Subsurface Structural Defects Detection in Concrete Bridge Decks. Infrastructures, 7(9), 107.
- Besharatian, B., & Dorafshan, S. (2022, June). Non-Contact Bridge Deck Evaluation Using Infrared Thermography, A Pipeline for Data Annotation. In 2022 International Conference on Unmanned Aircraft Systems (ICUAS) (pp. 1389-1396). IEEE.
- Ichi, E., & Dorafshan, S. (2022). Effectiveness of infrared thermography for delamination detection in reinforced concrete bridge decks. Automation in Construction, 142, 104523.
- Lavadiya, D. N., & Dorafshan, S. (2023). Deep learning models for analysis of non‐destructive evaluation data to evaluate reinforced concrete bridge decks: A survey. Engineering Reports, e12608.
- Ichi, E., & Dorafshan, S. (2024). Evaluation of Infrared Thermography Dataset for Delamination Detection in Reinforced Concrete Bridge Decks. Applied Sciences, 14(6), 2455.
- Yu, Y., Rashidi, M., Dorafshan, S., Samali, B., Farsangi, E. N., Yi, S., & Ding, Z. (2024). Ground penetrating radar-based automated defect identification of bridge decks: a hybrid approach. Journal of Civil Structural Health Monitoring, 1-23.
Presentations
Final Report
North Dakota Department of Transportation
Contact
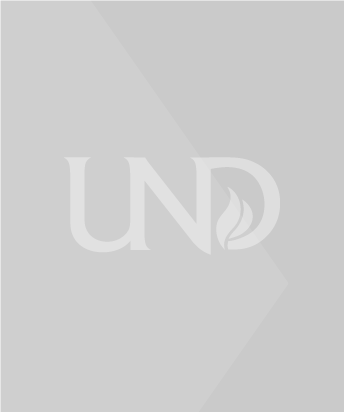
- dalton.l.reitz@UND.edu
- 701.777.3564
-
Upson II Room 260M
Grand Forks ND 58202-8115
The mission of the Center for Transformative Infrastructure Preservation and Sustainability (CTIPS) is to revolutionize the preservation of existing transportation systems through the integration of advanced sensing technologies and automation in data collection and analysis. The Center's research will also address systemic equity issues in the region, especially those stemming from the relatively poor quality of Tribal and rural roads.
Consortium Members
- Colorado State University
- Fort Lewis College
- North Dakota State University
- South Dakota State University
- United Tribes Technical College
- University of Colorado Denver
- University of Denver
- University of North Dakota
- University of Utah
- University of Wyoming
- Utah State University
Resources for CTIPS Researchers
Contact UND CTIPS Director
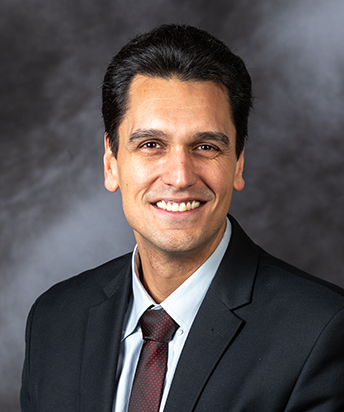
- sattar.dorafshan@UND.edu
- 701.777.0585
-
Upson Hall I Room 218
Grand Forks ND 58202-8115